Digital identities are transforming the daily lives of citizens worldwide, with strategies aimed at building a more secure and inclusive society.
The impact of deepfakes on the financial sector: how AI poses a threat to your company
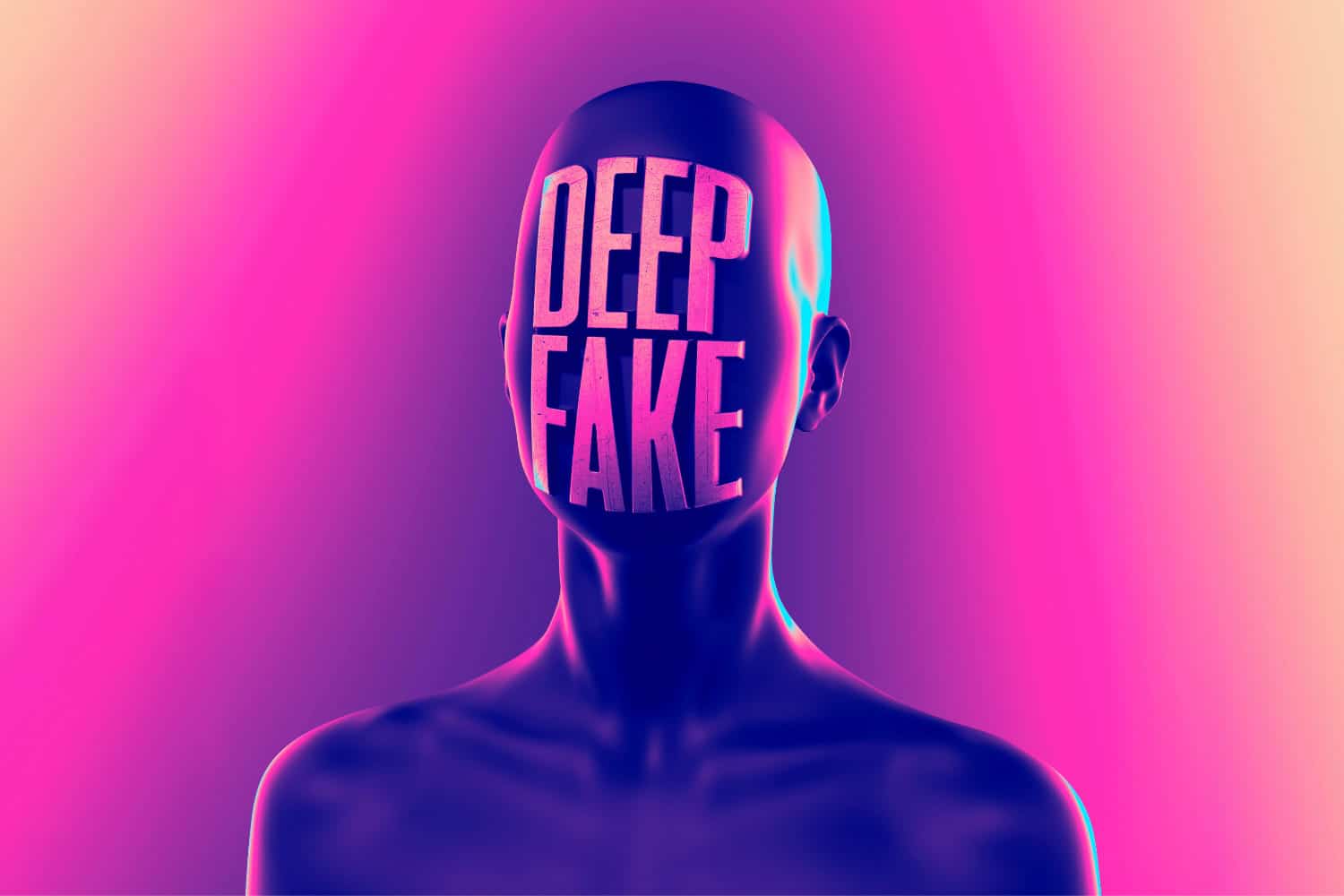
The controversial technique known as deepfake raises questions about the security of digital identification processes. To combat its negative effects, technology is striving to refine its detection mechanisms.
D
eepfakes have gained prominence for their astonishing ability to swap or replace faces in videos and images, deceiving viewers into believing that a person has said or done something that never took place. However, according to a study published in 2022, 70% of people worldwide do not know what this phenomenon entails, making it essential to engage in extensive educational and protective efforts.
This cutting-edge technology goes beyond simple facial swapping, as it can convincingly alter expressions, lip movements, and even the voice to create an authentic yet artificial representation of a person.
Deepfakes have various objectives. While they offer artistic and educational potential, cybercriminals have not hesitated to exploit their capabilities for purposes ranging from political and social manipulation to identity theft for illicit actions (financial fraud, illegitimate access to goods and services, etc.).
Creating a deepfake involves various techniques, from sophisticated methods leveraging the most advanced technology to analogue procedures attempting to alter the faces or identifications presented before the camera.
Deepfakes are closely related to synthetic identities: fictional or computer-generated digital profiles that can appear and interact like real people, even though they do not actually exist in the physical world. To make the result as convincing as possible, they are typically constructed from real information, much like a collage. Within this broader concept of fabricated digital identity, they can be used to create highly elaborate and realistic content.
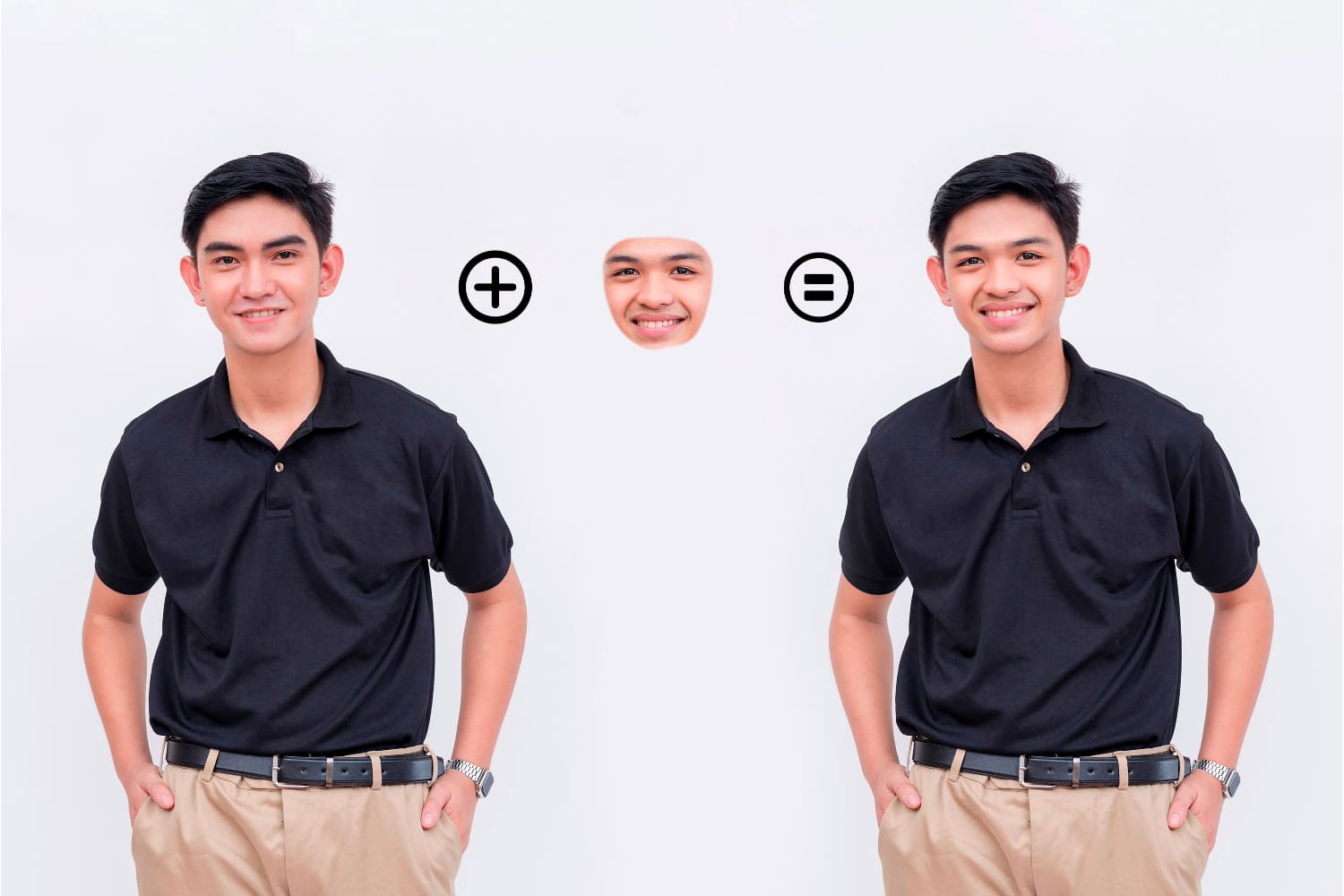
Video identification processes at risk
Deepfake technology can be so perfect that it may surpass video identification technology unless there is a solution focused on precise detection. This ability to manipulate or overlay images, videos, or audio from various sources is supported by the most advanced artificial intelligence.
Deepfakes rely heavily on deep learning techniques, particularly what is known as Generative Adversarial Networks (GANs), which generate synthetic material. These sophisticated algorithms give rise to deceptive content, astonishingly realistic in appearance, perpetuating the challenge of distinguishing genuine visual and auditory information from artificial.
GANs are a technique built upon two neural networks that compete against each other, a generator and a discriminator. The generator creates fake images, and the discriminator attempts to differentiate between real and fake ones. As both networks train, the generator improves its ability to create convincingly real-looking images, while the discriminator becomes more effective at detecting forgeries. This competition between the networks allows deepfakes to become increasingly convincing.
Although most deepfakes are created using deep learning algorithms and advanced software, it is also possible to craft video manipulations manually or analogously. In this more traditional approach, techniques like makeup, prosthetics, and visual effects can be used to achieve realistic transformations in the physical world, which can later be utilized in video identification or KYC procedures.
Through meticulous skills and creativity, experts achieve astounding results without relying on technology. This approach combines artistic prowess with manual ingenuity, underscoring that audiovisual manipulation is not confined solely to the digital realm, but can also be found at the intersection of art and craftsmanship.
A meticulous process to manufacture synthetic identities
The boundaries of this technique extend in complex ways into the creation of synthetic content. From voice manipulation to real-time recreation, and even building from authentic human traits, each step in the evolution of deepfakes reveals great depth.
- Images. Almost anyone can produce the deepfake images that we often encounter on the Internet. Individuals can take and use endless photos of public figures online. While creating deepfake videos can be easily done with applications and programs, the same can be achieved with images. Editing software puts faces on different bodies, much like in video editing. This is often used to present identity documents that appear official.
- Audio. Software programs use algorithms through an existing voice clip and create an entirely new one saying something different, mimicking voices, or including accents or tone. The more recordings available of the person being copied, the more accurate the creation of the audio deepfake will be.
- Real-time or live. Hackers attempt to bypass security measures (e.g., biometric parameter analysis or video identification) and complete an authentication or digital onboarding process during a live broadcast.
- From real faces. As mentioned earlier, deepfakes can be constructed from real information in a complex process that involves the following steps:
- Data collection, gathering fragments of real faces that will be used as training data for the AI.
- Feature extraction, using facial recognition algorithms to extract distinctive biometric features.
- AI model generation, using neural networks, such as GANs, trained with the collected fragments of real faces to generate images that are indistinguishable from real ones.
- Alignment and mapping, positioning the fragments of real faces in the target video. The AI used in the process identifies and fits the facial features in the target image, achieving a coherent and realistic appearance.
- Deepfake generation. The AI model generates a new video that combines the attributes of the real face with the original video.
- Improvement and refinement. The quality of the deepfake can be enhanced through post-processing techniques.
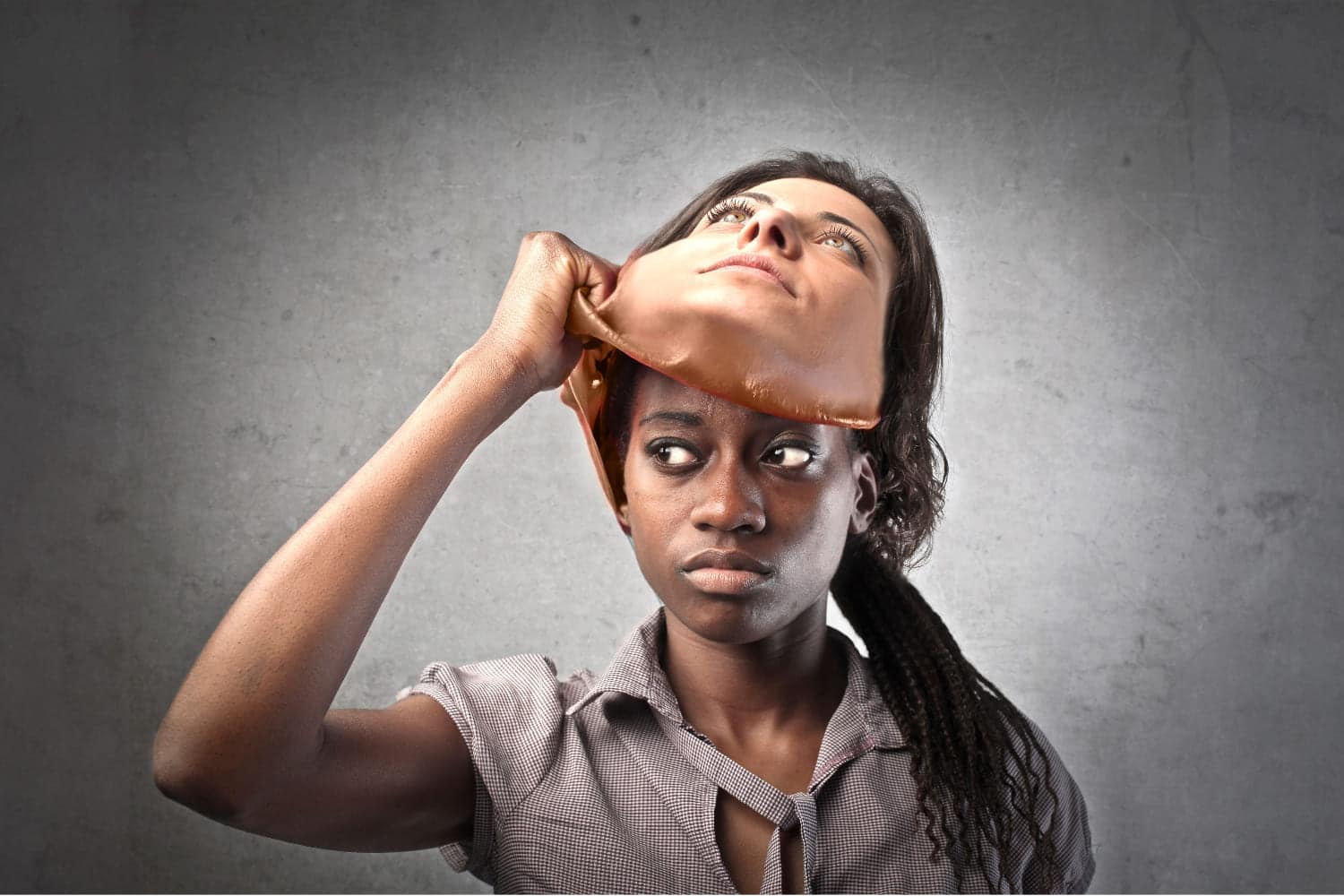
Advanced solutions for the most sophisticated fraud
In light of this scenario, it is necessary to create solutions that protect people’s identities and strengthen video verification, KYC, and KYB processes. Cloud-based deepfake detection is an advanced technology built on robust recognition capabilities for both video and image content. It can function independently as a standalone process or seamlessly integrate with others, such as digital identity authentication or video identification. The detection focuses on comprehensively addressing all facets of a deepfake procedure.
These procedures employ advanced algorithms to analyze the likelihood of identity theft or impersonation using real people images. They also assess the integrity of video content to identify any face manipulations and determine whether a face in an image is real or generated through GAN.
Deepfake detection is not only useful for the identification and digital onboarding process but also for fraud prevention and overall security.
TrustCloud’s strong proposition
The technology presented by TrustCloud through its platform can be applied both in real-time identification processes and in retrospective analysis of historical data. It requires user consent due to the use of biometric data in the process, complying with the strictest privacy standards.
In addition to deepfake detection in images and videos, it is important to note that TrustCloud’s solution also covers audio content. The technology is based on highly trained artificial intelligence models that have been taught to recognize patterns and characteristics of deepfakes, ensuring high detection accuracy.
TrustCloud’s tool is backed by a team of artificial intelligence and cybersecurity experts who are constantly working on the latest trends and techniques in deepfake generation, ensuring that the solution is always up-to-date and ready to face new challenges.
Aside from its usefulness in the identification and user authentication process, deepfake detection technology can be used in other scenarios such as fraud prevention in financial services, media integrity protection, and enterprise security.
This innovative and advanced solution is sure to make a difference in the fight against digital counterfeits and provide greater security and confidence to customers, positioning TrustCloud as a leader in this type of detection technology.
A multifaceted challenge: state-of-the-art technology and compliance
The use of deepfakes poses significant ethical challenges, such as the spread of false information, loss of trust in visual media, and invasion of privacy. There is a clear need for robust regulatory and ethical guidelines to mitigate the potential harm of these technologies and to ensure their responsible and beneficial use. Society must carefully consider the legal and ethical boundaries to balance creative innovation with the prevention of abuse and the protection of individuals and communities. As this phenomenon continues to expand, it is a wise stance to choose technological providers that ensure account openings, onboarding processes, and identity verification services backed by robust deepfake detection solutions.
This Post Has 0 Comments